👋 Hey, I’m Lenny and welcome to a 🔒 subscriber-only edition 🔒 of my weekly newsletter. Each week I tackle reader questions about building product, driving growth, and accelerating your career. For more: Best of Lenny’s Newsletter | Hire your next product leader | Podcast | Lennybot | Swag
You’re either building AI into your product now or you will be soon. And you’re probably already swimming in advice on the subject. But most of the advice out there is full of big, lofty ideas and light on tactics rooted in actual experience that you can implement today. So I teamed up with frequent collaborator Kyle Poyar to interview more than 20 successful builders and founders—people who have learned about building AI products the hard way—to share their biggest surprises and counterintuitive lessons. Many of these insights surprised me and got me thinking in a different way. I hope they will for you too.
Let me guess: You have a high-priority project on your roadmap right now to add (more) AI features to your product.
You’re in good company. A recent survey by Emergence Capital found that 60% of companies have already integrated generative AI into their products, and another 24% have it on their roadmap. AI is quickly eating the world.
Unfortunately, many of these efforts will end in failure. Most early AI apps have a “tourist” problem: they get a lot of traction quickly but have shockingly low retention and engagement. And according to that Emergence survey, two in five gen AI products still haven’t made a single dollar despite companies spending millions (or even billions) to build and support them. The goal of this post is to help you avoid wasting your precious time and resources by pointing your team in the wrong direction.
I polled more than 20 of the sharpest AI product builders asking them for the most counterintuitive and surprising things they’ve learned about building AI into their products. These leaders have built many of today’s most loved and successful AI products, including products at Adobe, GitHub, Intercom, Perplexity, Canva, Runway, HeyGen, and Superhuman. Here’s what I learned:
“It takes time to think AI-native. The first-pass product is often a bolt-on or simple chat experience. The high-value experience is a deeper rethink once you have played with the technology, understood what it really provides more deeply, and then integrated it into a key part of your product experience.”
—Elad Gil, technology entrepreneur and investor
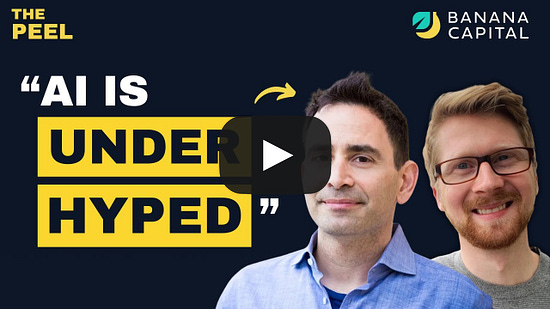
“It’s actually easier and safer for startups to work on hard problems, problems that cannot quite be solved with today’s foundation models. We’re excited about riding the capability curve of improving models, instead of fighting that progress.”
—Sarah Guo, startup investor and founder at Conviction
“Over the past 10 years, for most companies (with the exception of some hard-infra projects), it is assumed that what you want to build can be fairly easily built. You start by deeply understanding the customer problem and opportunity, design what you believe to be a great solution, and build it. AI is different.
With AI, it is wholly unclear if something is possible to build. And when it is built, it is wholly unclear if it is any good, even if it appears to be good. Often the best place to start projects is by asking, ‘What is technologically possible?’ and prototyping. This is a big mindset shift for anyone who has built software for the past decade and followed standard best practice.”
—Paul Adams, chief product officer at Intercom
“Demo value isn’t user value. Building a cool AI demo doesn’t mean we have a product that customers love and is useful.”
—Joshua Xu, co-founder and CEO at HeyGen
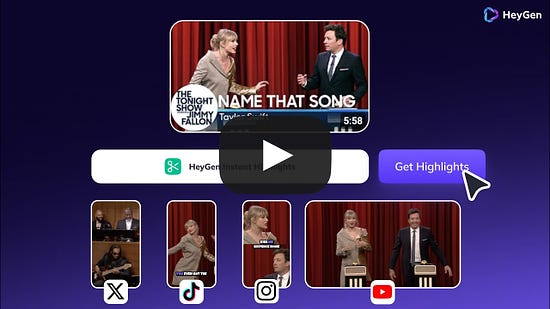
“I spend a lot of time thinking about adoption curve segmentation—identifying who adopts a new product quickly and who does not, and what distinguishes these groups. Historically, I’d focus on understanding the value a new product provides to people with different functional needs. AI changes this dynamic because the most meaningful segmentation often depends on attitudes toward the technology itself: AI embracers versus AI skeptics.
Many have discussed the AI ‘phantom PMF’ phenomenon, where novelty-driven acquisition leads to a steep churn cliff, but the reverse is also true. I frequently speak with customers who reject AI products that meet their needs simply because they don’t trust or want to embrace AI. With the right messaging and onboarding, these skeptics can become superusers! But they behave very differently from AI embracers. I sketched this out in the chart below.
As a result, I’ve had to rethink our user testing approach from the ground up. When testing AI products, I focus on the following:
Longitudinal validation: Are we testing for a long enough period to understand how engagement changes once the novelty wears off?
High-touch testing: Are we staying close enough to users to understand how their attitudes, which drive engagement patterns, change daily? We’re experimenting with user Slack groups instead of traditional surveys and one-on-one qualitative interviews.
Attitudinal segmentation: Are we including both AI embracers and AI skeptics in our early testing groups? Crucially, are we segmenting them carefully to avoid averaging out their engagement and creating ‘tepid tea’—a product that satisfies no one?”
—Hilary Gridley, head of core app product at WHOOP
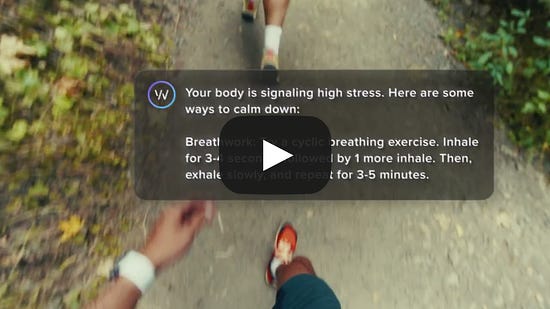
“Building fantastic product experiences hasn’t gotten easier with AI. Sci-fi capabilities of the models are inspiring, but that’s not what makes AI products great. Good old-fashioned product engineering does. This means homing in on real user pain points, iterating closely with customers, and holding a high bar for a delightful user experience.”
—Caitlin Colgrove, co-founder and chief technology officer at Hex
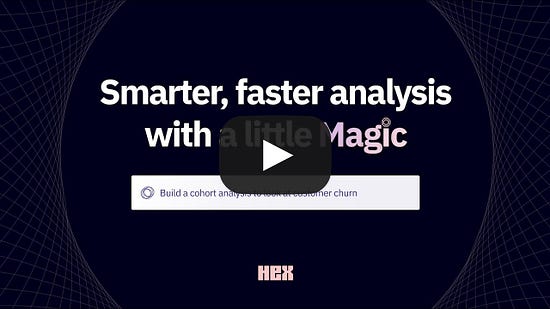
“Most people think about AI-assisted services in terms of the model quality, but model quality is just a tiny piece of the total product. It turns out that post-processing filters, contractual guarantees, data privacy, feedback loops, observable human impact, etc. are all far more important. In other words, building AI products looks a lot like building products.”
—Ryan J. Salva, vice president of product at GitHub
“The promise of AI (LLMs in particular) is to create anything for you with just a few words. But as we have seen over the past decade with Canva, when you give people the power to do anything, it can be pretty daunting and they don’t know where to start. So—just as with the very first version of our design tool—giving people the right starting points and confidence to utilize AI is a crucial part of delivering a great AI product.
The evolution of our Magic Media feature is a great example of this. Text-to-image is a magical piece of technology when you know what image you want and how to describe it. But most people don’t have the right vocabulary to properly explain what they’re looking for; or even worse, they don’t know what they’re looking for!
Our iterations on Magic Media have lessened that fear-inducing empty prompt box and introduced more visual options to guide you to a great image, as well as help to get people prompting in the right way. We’re also focusing on what happens after that generation—how you tweak and adjust what an AI has given to be exactly what you want.
All of this underscored to us that AI tools require a combination of intuitive product design and broader, ongoing education to support these behavior shifts. You can't ‘flip the switch’ with AI—society is in the midst of change at a cultural level, but well-built products can support this shift.”
—Cameron Adams, co-founder and chief product officer at Canva
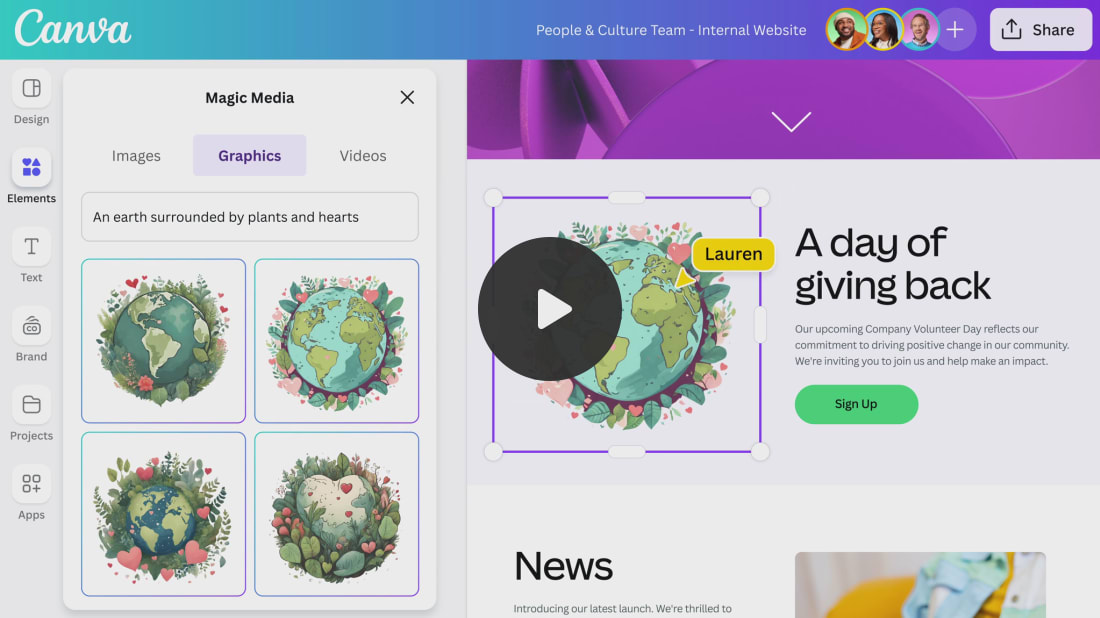
“Experimenting to find the right UI/UX for an AI feature can have an equally big impact on conversion metrics as research updates to the AI model itself. The right UX doesn’t just make new model capabilities more discoverable—it actually improves the conversion for users who use that capability, even if they were already using the capability anyway in the original UI.”
—Joel Kwartler, head of product at Runway
“The data and the interfaces may become more important than the models themselves, which are becoming increasingly commoditized, available via open source, and pushed to the edge (we’ll be running many models locally on devices within a few years). The AI products I am most excited about leverage a proprietary or uniquely structured set of data—for which they have a license to use rather than scrape it—and a superior interface that transforms an antiquated workflow.
What are the implications of this? Companies that have or really understand data in a deep vertical will have an advantage. And designers will be more important than ever before, imagining entirely new ways to transform the interfaces of our everyday work and life with the superpowers of AI.”
—Scott Belsky, chief strategy officer and executive vice president (EVP) of design and emerging products at Adobe; founder of Behance