Newsletters From:
Home
DeepLearning.AI | Andrew Ng | Join over 7 million people learning how to use and build AI through our online courses. Earn certifications, level up your skills, and stay ahead of the industry.
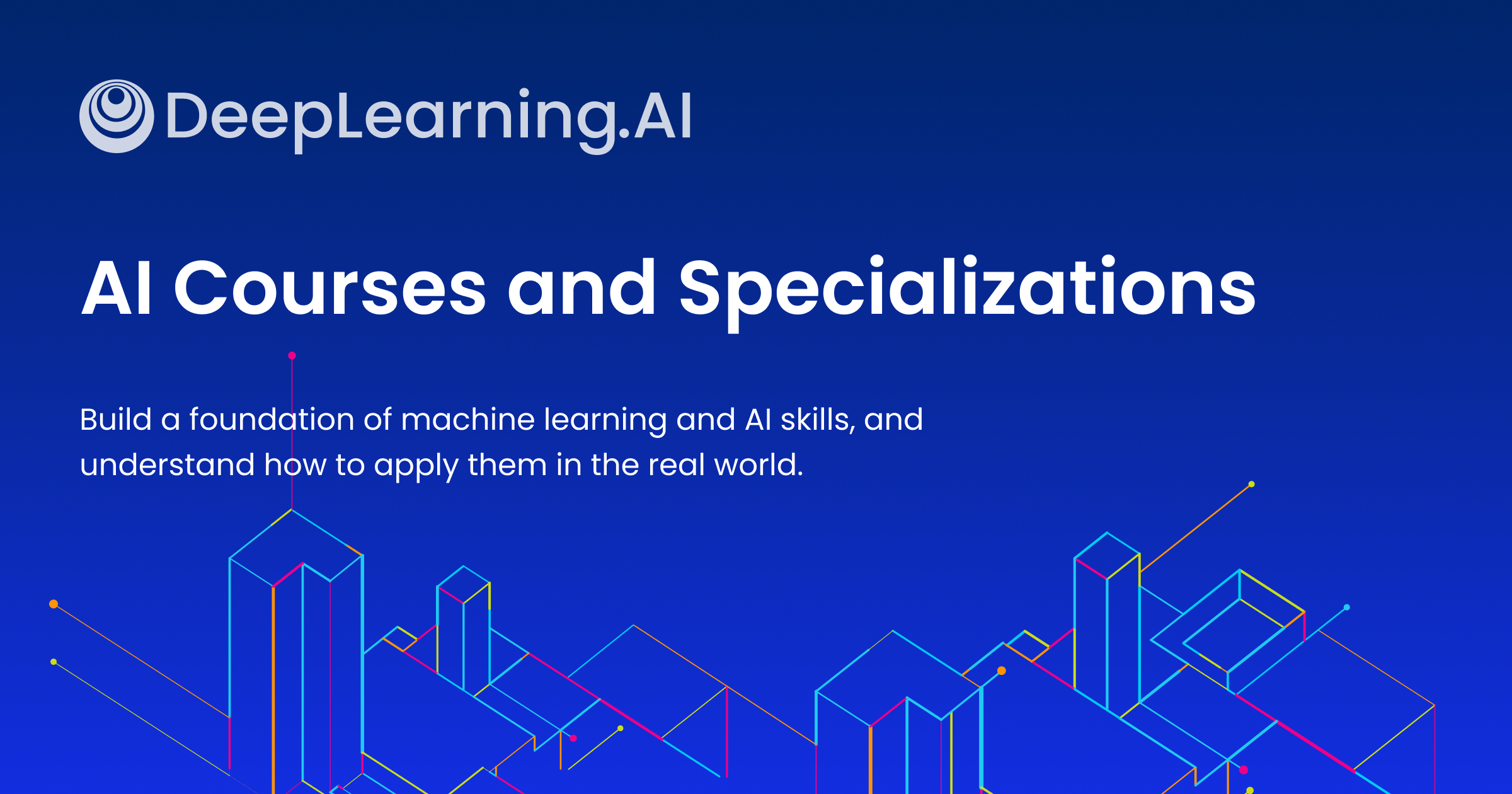
Hallucination Detector, Battle of the Image Generators, How Open Are Open Models?, Copyright Claim Fails Against GitHub
07-17-2024
Dear friends,
“Democracy is the worst form of government, except for all the others,” said Winston Churchill. Last week’s shocking attempt to assassinate former President Trump was a reminder that democracy is fragile.
But zooming out to a macro view,
I’m glad last week’s assassination attempt failed, just as I’m glad the January 6 insurrection at the U.S. Capitol failed. Both events were close calls and resulted in tragic loss of human life. Looking into the future, in addition to specific applications that strengthen elements of democracy, I hope we keep on promoting widespread access to technology. This will enhance fairness and the ability of individuals to vote wisely. That’s why democratizing access to technology will help democracy itself.
Keep learning! Andrew
A MESSAGE FROM DEEPLEARNING.AIEnhance your software-development workflow with our new course, “Generative AI for Software Development.” Learn how to use generative AI tools to boost efficiency, improve code quality, and collaborate creatively. Pre-enroll today and be the first to join when the course goes live
News
Copyright Claim Fails in GitHub CaseA judge rejected key claims in a lawsuit by developers against GitHub, Microsoft, and OpenAI, the first decision in a series of court actions related to generative AI. What’s new: A U.S. federal judge dismissed claims of copyright infringement and unfair profit in a class-action lawsuit that targeted GitHub Copilot and the OpenAI Codex language-to-code model that underpins it. The case: In November 2022, programmer Matthew Butterick and the Joseph Saveri Law Firm filed the lawsuit in U.S. federal court. The plaintiffs claimed that GitHub Copilot had generated unauthorized copies of open-source code hosted on GitHub, which OpenAI Codex used as training data. The copies allegedly infringed on developers’ copyrights. The defendants tried repeatedly to get the lawsuit thrown out of court. In May 2023, the judge dismissed some claims, including a key argument that GitHub Copilot could generate copies of public code without proper attribution, and allowed the plaintiffs to revise their arguments. The decision: The revised argument focused on GitHub Copilot’s duplication detection filter. When enabled, the filter detects output that matches public code on GitHub and revises it. The plaintiffs argued that the existence of this feature demonstrated GitHub Copilot’s ability to copy code in OpenAI Codex’s training set. The judge was not persuaded.
Yes, but: The lawsuit is reduced, but it isn’t finished. A breach-of-contract claim remains. The plaintiffs aim to show that OpenAI and GitHub used open-source code without providing proper attribution and thus violated open-source licenses. In addition, the plaintiffs will refile their unjust-enrichment claim. Behind the news: The suit against Github et al. is one of several underway that are testing the copyright implications of training AI systems. Getty Images, Authors’ Guild, The New York Times, and other media outlets along with a consortium of music-industry giants have sued OpenAI and other AI companies. All these cases rest on a claim that copying works protected by copyright for the purpose of training AI models violates the law — precisely what the plaintiffs failed to show in the GitHub case. Why it matters: This lawsuit specifically concerns code written by open-source developers. A verdict could determine how code can be used and how developers can use generative AI in their work. However, it has broader implications. (Note: We are not lawyers and we do not provide legal advice.) This dismissal is not a final verdict, but it suggests that AI developers may have a broad right to use data for training models even if that data is protected by copyright. We’re thinking: Broadly speaking, we would like AI to be allowed to do with data, including open source code, anything that humans can legally and ethically do, including study and learn. We hope the judge’s decision gives AI developers clarity on how they can use training data, and we hope it assures all developers that it’s ethical to use code-completion tools trained on open-source code.
How Open Are Open Models?The word “open” can mean many things with respect to AI. A new paper outlines the variations and ranks popular models for openness. What’s new: Researchers at Radboud University evaluated dozens of models billed as open by their developers. They plan to keep their analysis of language models updated here.
Results: Of the language models, OLMo 7B Instruct from Allen Institute for AI scored highest with 12 open characteristics and 1 partially open characteristic (it lacked a published, peer-reviewed paper).
Behind the News: The Open Source Initiative (OSI), a nonprofit organization that maintains standards for open-source software licenses, is leading a process to establish a firm definition of “open-source AI.” The current draft holds that an open-source model must include parameters, source code, and information on training data and methodologies under an OSI-recognized license. Why it matters: Openness is a cornerstone of innovation: It enables developers to build freely on one another’s work. It can also lubricate business insofar as it enables developers to sell products built upon fully open software. And it has growing regulatory implications. For example, the European Union’s AI Act regulates models that are released under an open source license less strictly than closed models. All these factors raise the stakes for clear, consistent definitions. The authors’ framework offers clear, detailed guidelines for developers — and policymakers — in search of clarity.
Image Generators in the ArenaAn arena-style contest pits the world’s best text-to-image generators against each other. What’s new: Artificial Analysis, a testing service for AI models, introduced the Text to Image Arena leaderboard, which ranks text-to-image models based on head-to-head matchups that are judged by the general public. At the time of this writing, Midjourney v6 beats more than a dozen other models models in its ability to generate images that reflect input prompts, though it lags behind competitors in speed. How it works: Artificial Analysis selects two models at random and feeds them a unique prompt. Then it presents the prompt and resulting images. Users can choose which model better reflects the prompt. The leaderboard ranks the models based on Elo ratings, which scores competitors relative to one another.
Who’s ahead?: As of this writing, Midjourney v6 (Elo rating 1,176), which won 71 percent of its matches, holds a slim lead over Stable Diffusion 3 (Elo rating 1,156), which won 67 percent. DALL·E 3 HD holds a distant third place, barely ahead of the open-source Playground v2.5. But there are tradeoffs: Midjourney v6 takes 85.3 seconds on average to generate an image, more than four times longer than DALL·E 3 HD and more than 13 times longer than Stable Diffusion 3. Midjourney v6 costs $66 per 1,000 images (an estimate by Artificial Analysis based on Midjourney’s policies, since the model doesn’t offer per-image pricing), nearly equal to Stable Diffusion 3 ($65), less than DALL·E 3 HD ($80), and significantly more than Playground v2.5 ($5.13 per 1,000 images via the Replicate API). Behind the news: The Text to Image Arena is a text-to-image counterpart of the LMSys Chatbot Arena, which lets users write a prompt, feed it to two large language models, and pick the winner. imgsys and Gen-AI Arena similarly let users choose between images generated by different models from the same prompt (Gen-AI Arena lets users write their own). However, these venues are limited to open models, which excludes the popular Midjourney and DALL·E. Why it matters: An image generator’s ability to respond appropriately to prompts is a subjective quality. Aggregating user preferences is a sensible way to measure it. However, individual tastes and applications differ, which makes personalized leaderboards useful as well.
Hallucination DetectorLarge language models can produce output that’s convincing but false. Researchers proposed a way to identify such hallucinations. What’s new: Sebastian Farquhar, Jannik Kossen, Lorenz Kuhn, and Yarin Gal at University of Oxford published a method that indicates whether a large language model (LLM) is likely to have hallucinated its output. Key insight: One way to estimate whether an LLM is hallucinating is to calculate the degree of uncertainty, or entropy, in its output based on the probability of each generated token in the output sequences. The higher the entropy, the more likely the output was hallucinated. However, this approach is flawed: Even if the model mostly generates outputs with a uniform meaning, the entropy of the outputs can still be high, since the same meaning can be phrased in many different ways. A better approach is to calculate entropy based on the distribution of generated meanings instead of generated sequences of words. Given a particular input, the more likely a model is to respond by generating outputs with a variety of meanings, the more likely that a response to that input is a hallucination. How it works: The authors generated answers to five open-ended question-and-answer datasets using various sizes of Falcon, LLaMA 2-chat, and Mistral. They checked the answers for hallucinations using the following method:
Results: The authors measured the classification performance of their method using AUROC, a score between .5 (the classifier is uninformative) and 1 (the classifier is perfect). On average, across all five datasets and six models, the authors’ method achieved .790 AUROC while the baseline entropy achieved .691 AUROC and the P(True) method achieved .698 AUROC. P(True) asks the model (i) to generate up to 20 answers and (ii) whether, given those answers, the one with the highest probability of having been generated is true or false. Yes, but: The authors’ method fails to detect hallucinations if a model consistently generates wrong answers. Behind the news: Hallucinations can be a major obstacle to deploying generative AI applications, particularly in fields like medicine or law where missteps can result in injury. One study published earlier this year found that three generative legal tools produced at least partially incorrect or incomplete information in response to at least one out of every six prompts. For example, given the prompt, “Are the deadlines established by the bankruptcy rules for objecting to discharge jurisdictional,” one model cited a nonexistent rule: “[A] paragraph from the Federal Rules of Bankruptcy Procedure, Rule 4007 states that the deadlines set by bankruptcy rules governing the filing of dischargeability complaints are jurisdictional.” We’re thinking: Effective detection of hallucinations not only fosters trust in users — and thus potentially greater adoption — but also enables researchers to determine circumstances in which they occur, so they can be reduced in future models.
A MESSAGE FROM DEEPLEARNING.AIIn “Pretraining LLMs,” a short course built in collaboration with Upstage, you’ll learn about pretraining, the first step of training a large language model. You’ll also learn innovative pretraining techniques like depth upscaling, which can reduce training costs by up to 70 percent. Join today
Work With Andrew Ng
Join the teams that are bringing AI to the world! Check out job openings at DeepLearning.AI, AI Fund, and Landing AI.
Subscribe and view previous issues here.
Thoughts, suggestions, feedback? Please send to thebatch@deeplearning.ai. Avoid our newsletter ending up in your spam folder by adding our email address to your contacts list.
|
.png?width=1200&upscale=true&name=The%20Batch%20top%20banners%20(27).png)
07-17-2024
Hallucination Detector, Battle of the Image Generators, How Open Are Open Models?, Copyright Claim Fails Against GitHub
#hs_body #hs_cos_wrapper_main a[x-apple-data-detectors]{color:inherit !important;text-decoration:none !important;font-size:inherit !important;font-family:inherit !important;font-weight:inherit !imp
Read More